Machine learning is a subset of artificial intelligence that allows computers to learn from data and make predictions or decisions without requiring explicit programming. Machine learning can analyze massive amounts of medical data and provide insights into treatment plans, healthcare processes, and patient outcomes.
Machine learning in healthcare has recently emerged as one of the most popular buzzwords. But what exactly does machine learning in healthcare mean? What are benefits and application of machine learning in healthcare?
But first, let us look at some statistics and facts about machine learning in healthcare:
- The global AI in healthcare market is expected to reach $45.2 billion by 2026, with a compound annual growth rate (CAGR) of 44.9% between 2021 and 2026.
- Accenture estimates that critical clinical health AI applications have the potential to save the US healthcare economy $150 billion per year by 2026.
- According to Frost & Sullivan, by 2025, AI systems will be used in 90% of US hospitals and 60% of global insurance companies.
- According to a Science Direct report, around 86% of healthcare organizations use various machine learning solutions. Furthermore, over 80% of healthcare leaders have used artificial intelligence (AI) in some capacity.
What is machine learning in healthcare?
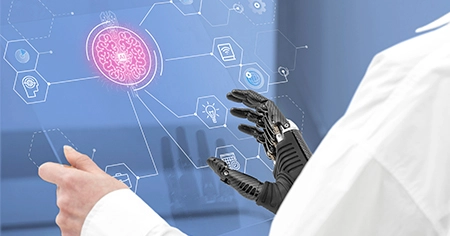
Machine learning in healthcare depends on gathering patient data. Machine learning algorithms can uncover patterns in these datasets using systems and tools to organize and classify the data. This allows healthcare professionals to detect new illnesses and predict the outcome of treatments.
The amount of patient data gathered in healthcare settings, especially across a state or country, is staggering. All medical devices and records can be integrated into a single network for efficient data management. This integration helps data scientists identify trends and patterns efficiently.
Why is Machine Learning Important in Healthcare Organizations?
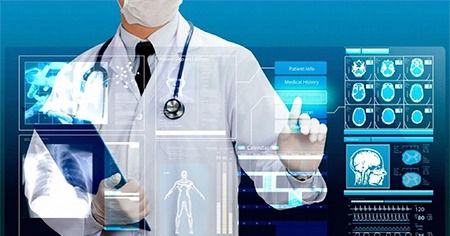
Machine learning algorithms are helpful in the healthcare industry because they can help us make sense of the massive amounts of healthcare data generated daily by electronic health records. Using machine learning in healthcare, such as machine learning algorithms, allows us to discover patterns and insights in medical data that would be impossible to find manually. This is especially crucial for specialized medical professions such as acupunture schools or massage therapy schools, which heavily depend on the anatomy of the body.
As machine learning in healthcare becomes more widely used, healthcare providers can take a more predictive approach to precision medicine, resulting in a more unified system with improved care delivery, better patient outcomes, and more efficient patient-based processes.
"Healthcare professionals commonly use machine learning for medical billing automation, clinical decision support, and developing clinical practice guidelines within health systems. Many practices also choose to outsource medical billing to specialized services, leveraging machine learning to enhance accuracy and reduce administrative burdens."
The complexity, lack of uniformity, ambiguity, and jargon of human language make communicating difficult. Healthcare machine learning frequently relies on artificial intelligence tools such as natural language processing programs to convert these documents into more analytic data.
Applications of Machine Learning in Healthcare:
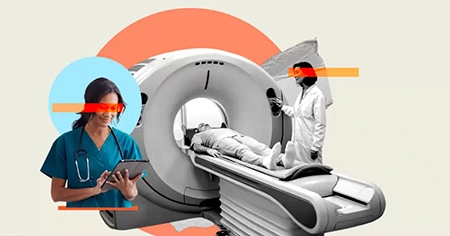
Machine learning in healthcare has ushered in a new era of innovation and transformation in medicine. These cutting-edge technologies use algorithms and data-driven insights to give healthcare professionals powerful diagnostic, treatment, and patient care tools.
So, how can machine learning be used in healthcare? Here are some of the most common uses of machine learning in healthcare. Let's look:
- Disease diagnostics and predictive analytics
Disease diagnosis and predictive analytics are among healthcare's most promising machine learning applications. According to Global Market Insights, disease diagnosis powered by AI and ML is expected to grow by more than 40%, surpassing the significant milestone of USD 2.5 billion by 2024.
Machine learning models can analyze patient data to identify early signs of cancer, diabetes, and heart disease. Early detection can significantly improve treatment outcomes and save lives.
For example, Algorithms can examine medical images such as X-rays and MRIs to detect anomalies that may indicate a disease.
Machine learning is also important in predictive analytics, which helps forecast disease trends and patient outcomes. Machine learning models can use historical data to predict disease outbreaks, chronic illness progression, and patient readmissions.
- Drug development and production
Machine learning plays a significant role in the early stages of drug development. This includes research technologies such as precision medicine and next-generation sequencing, which can help develop therapies for complex diseases.
This type of machine learning often uses unsupervised learning to detect patterns in data without making predictions. Microsoft's Project Hanover uses machine learning (ML) technologies for various purposes, including AI-driven cancer treatment approaches and drug combinations tailored for Acute Myeloid Leukemia (AML).
- Fraud detection and prevention
According to the NHCAA (National Health Care Anti-Fraud Association), healthcare fraud costs billions annually, accounting for 3 to 10% of healthcare expenditures. This translates into an annual loss of approximately $300 billion.
Machine learning in healthcare can detect invalid insurance claims before they are paid and expedite the approval, processing, and payment of valid ones. In addition to detecting insurance fraud, machine learning applications in healthcare can help prevent patient data theft.
- Personalized treatment plans
Every patient is unique, and machine learning enables doctors to tailor treatment plans to their specific characteristics and medical history. This personalized approach produces more effective treatments with fewer side effects.
For example, machine learning can use genetic information to recommend the best medication and dosage for a patient, reducing the risk of adverse reactions.
- Remote patient monitoring and telemedicine
Machine learning can use data from wearable devices and remote monitoring systems to track patients' health and identify potential problems before they worsen. This enables healthcare providers to provide timely interventions, thereby improving patient outcomes. Telemedicine platforms such as Teladoc and Doctor on Demand use machine learning algorithms to assess patient data and recommend appropriate treatment.
- Medical imaging diagnosis
Machine learning and deep learning are both responsible for the groundbreaking technology known as computer vision. This has received approval from Microsoft's InnerEye initiative, which focuses on image diagnostic tools for image analysis. As machine learning becomes more accessible and explanatory, more data sources from various medical images will be integrated into this AI-driven diagnostic process.
- Clinical Trials and Research
Machine learning has numerous applications in clinical trials and research. These costly and time-consuming trials can benefit from ML's predictive analytics to identify suitable candidates by drawing on various data sources, including previous doctor visits and social media. Machine learning tracks trial participants in real time maximize sample sizes and improves data accuracy using electronic records.
- Clinical Trials and Research
Machine learning has numerous applications in clinical trials and research. These costly and time-consuming trials can benefit from ML's predictive analytics to identify suitable candidates by drawing on various data sources, including previous doctor visits and social media. Machine learning tracks trial participants in real time maximize sample sizes and improves data accuracy using electronic records.
- Better Radiotherapy
Radiology is one of the most popular applications of machine learning in healthcare. Many discrete variables can arise during the medical image analysis process. Many lesions, cancer foci, and so on cannot be easily modelled using complex equations. Because ML-based algorithms learn from the many samples available, diagnosing and identifying variables becomes easier.
- Robot-Assisted Surgery.
ML-powered surgical robots have transformed surgical procedures in terms of precision and speed. These systems can perform complex surgical procedures while minimizing blood loss, side effects, and pain. Furthermore, postoperative recovery is much faster and simpler.
- Machine Learning-based Behavior Modification
Behavioral modification is an essential component of preventive medicine. Since the introduction of machine learning in healthcare, numerous startups have emerged in cancer prevention and detection, patient treatment, and so on. Somatic is a B2B2C data analytics company that has created an ML-based app that recognizes gestures we make in our daily lives, allowing us to understand our unconscious behavior better and make necessary changes.
Benefits of Machine Learning Application In Healthcare:
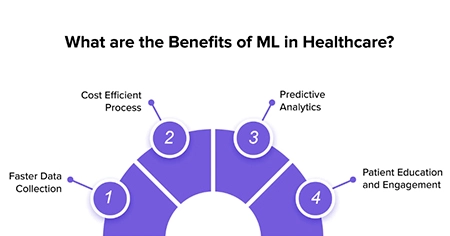
Machine learning has many benefits that could transform patient
care and healthcare operations. This cutting-edge technology
uses data-driven insights and algorithms to improve medical
decision-making, diagnose faster, optimize treatment plans, and
improve patient outcomes.
Here's a list of just a few of the benefits that machine
learning applications in healthcare can bring to healthcare
professionals:
- Improving diagnosis
Medical professionals can use machine learning in healthcare to create better diagnostic tools for medical image analysis. For example, in medical imaging (such as X-rays or MRI scans), a machine-learning algorithm can look for patterns that indicate a specific disease.
- Reduce Costs
Machine learning can help healthcare organizations save money by reducing manual labour and medical errors by automating routine tasks and increasing accuracy. Furthermore, machine learning can identify patients at risk of developing chronic conditions, allowing for early intervention and preventive care while potentially lowering long-term healthcare costs.
- Developing new treatments/drug discovery / clinical trials
Healthcare organizations and pharmaceutical companies can also use a deep learning model to identify relevant information in data, which could lead to drug discovery, drug development, and new disease treatments. For example, machine learning could be used in healthcare to analyze data and medical research from clinical trials to discover previously unknown drug side effects.
- Predictive healthcare
Machine learning's ability to analyze large datasets and identify patterns makes it extremely useful in predictive healthcare. Predictive models can predict disease outbreaks, readmissions, and equipment failures. This proactive approach enables healthcare institutions to implement preventive measures, which improves overall patient care and safety.
- Detect Fraud
According to the Justice Department, approximately 3% of healthcare claims in the United States are false. Every year, this results in a $100 billion loss in revenue. The healthcare industry could speed up the approval, processing, and payment of valid claims by employing machine learning models to detect fraudulent ones before they are paid.
- Automate and optimize processes.
Healthcare professionals may benefit from using machine learning to automate and optimize procedures. In practice, machine learning (ML) can automatically process massive amounts of medical data and generate personalized treatment plans for patients, saving time and effort that would otherwise be spent improving patient care.
Challenges and limitations of machine learning in healthcare:
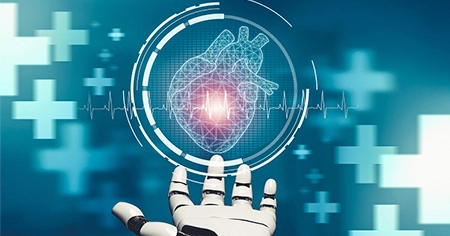
Machine learning in healthcare faces multiple challenges, including:
- Data privacy
Data privacy is critical in healthcare because it contains sensitive patient information. When implementing machine learning, prioritize data security and patient privacy.
- Lack of interoperability
Interoperability is difficult because healthcare systems frequently use different data formats and standards. Machine learning solutions must integrate seamlessly with various systems to be effective. Lack of interoperability impedes the exchange of patient data between healthcare providers, resulting in fragmented care and potential errors.
- Unstructured Data
The final issue facing machine learning healthcare is unstructured data. Medical data is frequently unstructured, which makes machine learning difficult. Effective methods of structuring and organizing medical data for machine learning must be developed to overcome this barrier.
- Skill gaps and training
Healthcare professionals require training to use machine
learning tools effectively effectively. Bridging the skills gap
between technology and healthcare is critical for successful
implementation.
Healthcare providers like doctors, nurses, and administrators
must learn to work with machine-learning algorithms and
interpret their results.
- Bias and fairness of machine learning
Machine learning models can inherit biases from training data, resulting in unequal treatment of specific patient groups. Bias in healthcare AI can lead to disparities in diagnosis and treatment, which harms vulnerable populations. Addressing these biases and ensuring fairness in healthcare AI remains a significant concern.
The future of machine learning in healthcare:
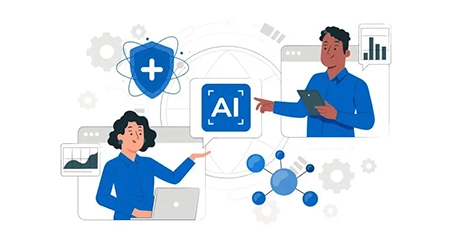
The future of machine learning in healthcare is promising and moving towards delivering truly proactive and predictive healthcare systems. However, the journey is complex and lengthy and requires multiple stakeholders, such as technology companies, healthcare providers and governments. It also helps to improve the clinical practice, pharmaceutical operations and patient experience.
The technology analyses hundreds of data points, personalizes treatment plans, predicts risks and outcomes, and performs various other tasks. AI and machine learning in healthcare also provide earlier warnings for conditions such as seizures and sepsis, necessitating extensive analysis of highly complex datasets.
Professionals can use machine learning and nanotechnology to improve medicine delivery in the coming years. Machine learning in healthcare can also enable "virtual biopsies" and advance radiomics, an innovative field. By focusing on even the most minor treatment details, ML-powered technologies can assist doctors in reducing risk during operations. Furthermore, programmed robots may eventually help doctors in the operating room.
Healthcare machine-learning solutions can benefit doctors, clinicians, researchers, and patients. Every day, technology makes a breakthrough, creating a new application that solves a real problem or establishes a milestone. The advancement of machine learning in healthcare continues to accelerate, and it appears to reach new heights in the coming years.
Conclusion
Machine learning is revolutionizing healthcare, with advances ranging from improved health insurance processes to robot-assisted surgery innovations and virtual nursing. It impacts disease diagnosis, personalized medicine, drug development, clinical trials, medical imaging, and improving care efficiency and personalization.