This transition is primarily driven by the integration of cutting-edge technology, with artificial intelligence (AI) taking the lead as a revolutionary force with the potential to reshape healthcare as we know it. As healthcare practitioners and institutions attempt to provide more precise diagnoses, individualized treatment plans, and streamlined administrative procedures, AI's role becomes clear and necessary.
AI, defined by its ability to mimic human cognitive functions and execute activities that previously required human intelligence, has great promise in healthcare software development companies. Machine learning algorithms, natural language processing, and computer vision are some AI techniques that allow healthcare systems to analyze large datasets, identify nuanced patterns, and derive actionable insights in real-time.
In this article, we will explore the innovations of software-defined networks and AI for cybersecurity in healthcare industry.
The following statistics of Cyberattacks says that:
- 50% more attacks each week in 2021 compared to 2020.
- The average overall cost of a data breach rose from $3.86 million to $4.24 million in 2021.
- 50% year-over-year increase in the number of cyberattacks
- Data breaches with longer reaction times (greater than 200 days) cost an average of $4.87 million, while breaches with shorter response times cost an average of $3.61 million.
- 400% rise year over year in phishing assaults.
- 93% of networks are believed to be vulnerable to cyberattacks.
- $458.9 billion planned cybersecurity spending by 2025.
What is Software-Defined Networking (SDN)?
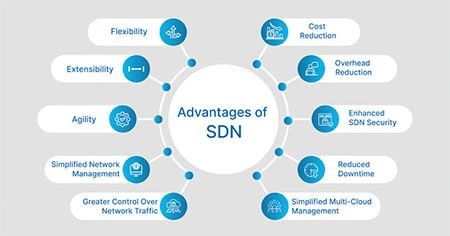
Software-defined Networking (SDN) is a novel network administration method that separates the control and data planes.
In simple terms, SDN enables network administrators to control and optimize network resources through software rather than hardware. This allows for increased flexibility, scalability, and control over network operations.
The Growing Importance of Software Defined Networks and AI for Cybersecurity in Healthcare Industry:
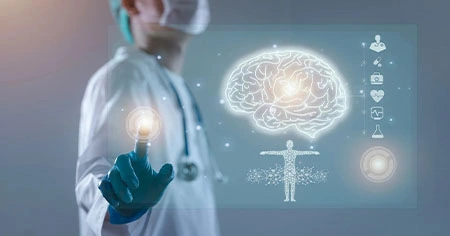
AI is becoming an important aspect of healthcare, from assisting doctors with diagnostics to organizing medical chores and discovering novel treatments. Due to the sensitivity of patient data, the healthcare industry has become a popular target for cybercriminals. Healthcare software development company hold huge amounts of personal information, including electronic health records (EHRs) and IoT devices, which might be exploited if not well protected.
AI methods like machine learning and deep learning can sift through large amounts of medical data to identify patterns and forecast what might happen. For example, AI can assist clinicians in detecting issues in medical pictures, allowing for faster and more accurate diagnoses. According to Frost and Sullivan's projections, AI is expected to improve patient outcomes by 30% to 40% while reducing treatment costs by 50%.
AI introduces advanced capabilities for detecting, analyzing, and responding to cyber threats. AI-powered systems use machine learning (ML) and data analytics to detect real-time trends, anomalies, and potential security flaws.
AI-powered virtual assistants and chatbots are being used to provide basic medical information, answer patient questions, and assess symptoms. They are important in appointment scheduling, medication reminders, and post-treatment follow-up. By improving patient engagement and healthcare accessibility, these AI-powered products help to provide a more seamless healthcare experience. Using natural language processing (NLP) technology, this automation reduces the workload on healthcare personnel, increases overall efficiency, and allows healthcare workers to devote more time and attention to patient care, maximizing their concentration and productivity.
While AI has the potential to revolutionize healthcare, the industry's rising reliance on digital infrastructure makes it vulnerable to cyber threats. The importance of patient information, research data, and patented medical technology makes healthcare organizations attractive targets for cybercriminals.
Key Features of SDN:
- Centralized management for easier configuration and monitoring.
- Dynamic resource allocation to meet network demands.
- Enhanced visibility and control over network traffic.
- In healthcare, SDN facilitates the secure and seamless exchange of sensitive data, ensuring compliance with regulations like HIPAA.
Innovations in Healthcare Cybersecurity with SDN and AI
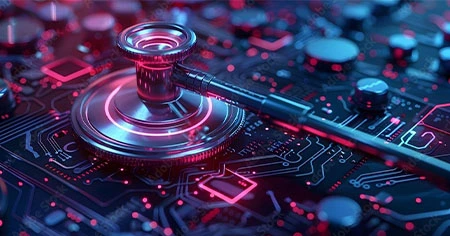
When combined, Software Defined Network and AI for Cybersecurity in Healthcare Industry offer several critical advantages:
Real-Time Threat Detection and Mitigation: SDN and AI collaborate to identify and neutralize threats in real time. SDN's centralized control allows rapid adjustments, while AI analyzes data to pinpoint anomalies and emerging threats.
Enhanced Data Encryption: Both technologies support advanced encryption protocols to protect patient data during transmission and storage.
Dynamic Access Control: AI-powered SDN systems enforce role-based access controls, ensuring only authorized personnel can access sensitive information.
Network Segmentation: SDN enables the creation of isolated network segments for critical healthcare systems, limiting the spread of cyber threats.
Proactive Risk Management: AI's predictive capabilities identify vulnerabilities before they can be exploited, allowing for proactive measures.
Improved Compliance: SDN and AI help healthcare software development companies adhere to strict regulatory standards by monitoring and documenting network activities.
Resilience Against Ransomware Attacks: AI algorithms analyze ransomware patterns, while SDN can quarantine infected systems, minimizing damage.
Pros and Cons of AI in Healthcare
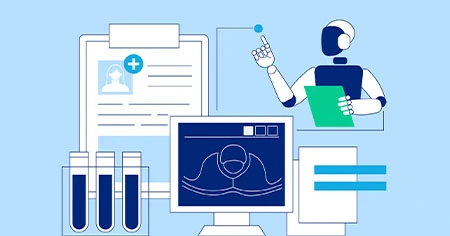
Artificial Intelligence (AI) is revolutionizing the healthcare industry by improving diagnostics, enhancing patient care, and streamlining operations. However, despite its transformative potential, AI also presents challenges and risks. Below is a detailed exploration of the pros and cons of AI in healthcare.
Pros of AI in Healthcare
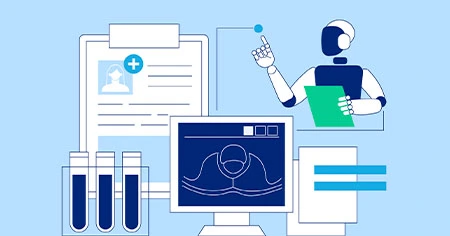
Enhanced Diagnostics and Early Detection
- Improved Accuracy: AI algorithms can analyze medical data (e.g., images, lab results) with high precision, often surpassing human capabilities.
- Early Detection: AI systems can identify patterns indicative of diseases like cancer or diabetes early, enabling timely intervention.
Personalized Medicine
- AI helps tailor treatments to individual patients by analyzing genetic, environmental, and lifestyle data, improving outcomes.
- Predictive analytics can suggest specific therapies based on patient profiles.
Operational Efficiency
- Automation of Administrative Tasks: AI reduces time spent on paperwork, scheduling, and data entry, allowing healthcare professionals to focus on patient care.
- Resource Management: AI optimizes staffing, inventory, and equipment usage.
Remote Patient Monitoring and Telehealth
- AI-powered tools allow continuous monitoring of patients with chronic conditions, reducing hospital visits.
- Virtual assistants and chatbots can provide basic healthcare guidance, improving accessibility.
Drug Discovery and Development
- AI accelerates the discovery of new drugs by analyzing vast datasets, reducing the time and cost associated with clinical trials.
- It can predict potential side effects or the effectiveness of new medications.
Reduced Costs
- AI can lower healthcare costs by improving efficiency, reducing errors, and enabling preventive care.
Improved Patient Outcomes
- AI enhances decision-making, leading to more effective treatments and better patient experiences.
Cons of AI in Healthcare
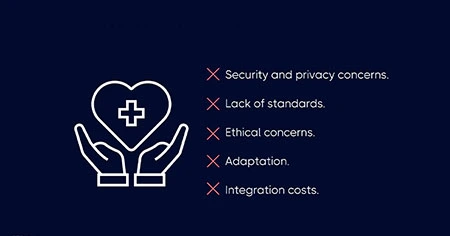
High Implementation Costs
- Initial Investment: Developing and deploying AI systems requires substantial financial resources.
- Maintenance and Upgrades: Ongoing costs for updates, repairs, and training staff add to the financial burden.
Data Privacy and Security Risks
- AI relies on vast amounts of sensitive patient data, making it a target for cyberattacks.
- Breaches could lead to significant legal and ethical consequences.
Bias and Inequality
- AI algorithms can inherit biases from training datasets, potentially leading to unequal treatment or misdiagnoses.
- Lack of diverse data can exacerbate health disparities among underrepresented populations.
Job Displacement and Resistance
- Automation of tasks may reduce the need for specific roles, causing job losses in administrative and diagnostic areas.
- Resistance to change from healthcare software development companies may slow AI adoption.
Reliability Concerns
- AI systems are not infallible; algorithms or data interpretation errors can lead to misdiagnoses or inappropriate treatments.
- More reliance on AI may erode critical thinking and decision-making skills among healthcare providers.
Regulatory and Ethical Challenges
- Developing consistent regulations to govern AI in healthcare is complex.
- Ethical dilemmas, such as decision-making accountability and patient consent, still need to be solved.
Interoperability Issues
- Integrating AI systems with existing healthcare infrastructure can be challenging.
- Lack of standardization across platforms may hinder seamless data exchange.